How smart cities can overcome ‘the data paradox’
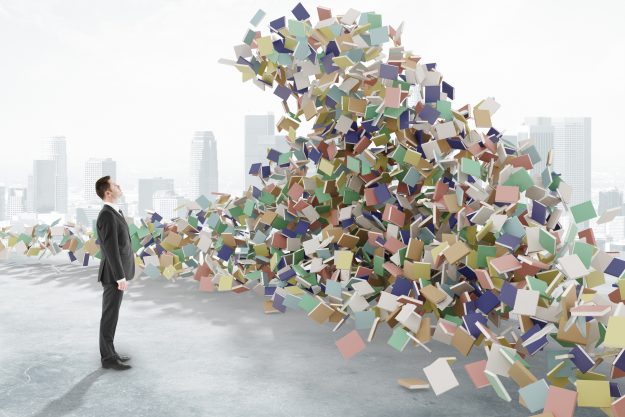
As cities increasingly try to operate in a smarter, more data-driven way, they are confronting a data paradox. On the one hand, cities have more and more terabytes of system-generated data than they know what to do with. On the other hand, they do not have enough of the right data to help them make important policy and budget decisions based on data instead of generalized assumptions. How can this be — how can cities both have too much data and not enough data at the same time?
To better understand this “data paradox,” which my colleague David D’Silva touched on in a recent opinion piece written for Smart Cities Dive , we need to segment the issue into three parts:
1. Too much generated data
In every major city in the United States, and beyond, there are millions of sensors producing a staggering amount of data every millisecond, second, minute, hour, and day. Consider traffic signal systems — a large city has thousands of signals, creating huge amounts of data every day, and that’s just for one metro-wide system. In many circumstances, that signal data is captured, stored and more or less forgotten. Often it is only when specific events like traffic backups activate the alert system in real time that the engineers interact with the data to gain insights about how well the system is functioning. This signal data is reviewed and properly stored, but it’s not often used for analysis to address recurring or future problems in a preventative, proactive analysis.
Cities face three challenges in activating insights and utility from this kind of generated data:
Storage – Each new sensor type often requires a new database, which is often subject to the city procurement process. These systems frequently do not talk to each other in ways that are useful or intuitive, making it virtually impossible to extract actionable insights from the information.
Staffing – A variety of budget constraints often push cities to employ consultants in lieu of hiring new staff that must be funded year over year. While this is a reasonable short-term solution it also means there is not long-term analysis capability for sustainability, nor the knowledge retention to recreate the analysis at some point in the future.
Procurement – “As a service” products are relatively new to the government space, and as these new cloud-based systems are implemented, governments often need to develop new policies and procedures to handle the purchase and maintenance of the changing technical infrastructure landscape.
2. Too little of the right data
In order to create a set of sustainable, equitable policies for cities, it is important to understand not just what people are doing today in terms of their transit and mobility patterns, but what they are trying to do and where they are trying to go. What is the ideal way for someone to get where they are going? These behavioral datasets are often complicated and expensive, if not impossible, to collect in a timely fashion that reflects the diversity of needs present in a city.
To conduct effective transportation planning, layering analysis is critical. People generally are trying to get to jobs, healthcare, education, services, and entertainment. But the right data is often not available to planners — they often don’t know where the jobs are or what services people are trying to access compared to where they live. They may be able to figure out how long it would take a given person to go from point A to point B, but understanding that the individual really wants to go to point C and then point D is beyond the scope of the datasets that are available to them. Outside of census data, transportation planners often don’t have real-time holistic insight across the city related to what jobs are available or under-filled, and where the folks that are qualified for those jobs live. One of the grand challenges for economic growth for cities is to connect individuals with the optimal job they are qualified for, but in many cases this data just does not exist or is not available to city and transportation planners.
3. A constantly evolving, massively complex data ecosystem
There is a third angle, where the data exists and is not too big or too small but may still be difficult to access. Within the city data ecosystem, there are a few different scenarios where the city agency does not have access to the data that is necessary to answer its questions. It is possible to access this kind of data, but it often requires lengthy and complicated data-sharing processes that have several hurdles, including:
Data discoverability/agency ownership – In some cases the data may exist, but it is unclear who owns it, or another city department may own it and be unwilling to share. Despite being “city data,” these silos often exist in a way that does not make it obvious how to share data to achieve a common solution (or how to maintain a shared data warehouse or analytics solution).
Jurisdictional ownership – Similarly, the on-the-ground reality may span multiple jurisdictions: for most transportation systems, the city, county, region, and state all have different oversight and ownership of the transportation options any one citizen may take in a given day. In this case, the data exists, but the complex partnerships required to build a cohesive picture often involve lengthy data sharing discussions.
Vendor ownership – For vendor-hosted solutions, the city may not own the data or be able to port the data to its analysis tools, which renders usage of the data with other city data sets difficult, if not impossible. Many vendors also require additional fees to extract data out of their systems.
Moving forward
This paradox has created a dilemma that cities must contend with. Some cities have decided to begin digging through the data at hand to gain insights from the data they already own, choosing not to wait for the identification and collection of user-centric data about transportation consumers’ actual behavior. Alternatively, the private sector can work closer with cities on this challenge. These conditions are not unique to the public sector, and in some instances, have already been solved in the private sector — there is an opportunity to use those solutions and approaches as a model for solutions in the city space.
While there is no magic answer to solve this kind of conundrum, I believe we need to begin a real and sustained effort that is intended to identify, develop, refine and deploy new data-driven solutions that will enable millions of citizens around the world in our major cities.