Virginia Tech data program boosts local agencies short on analytical resources
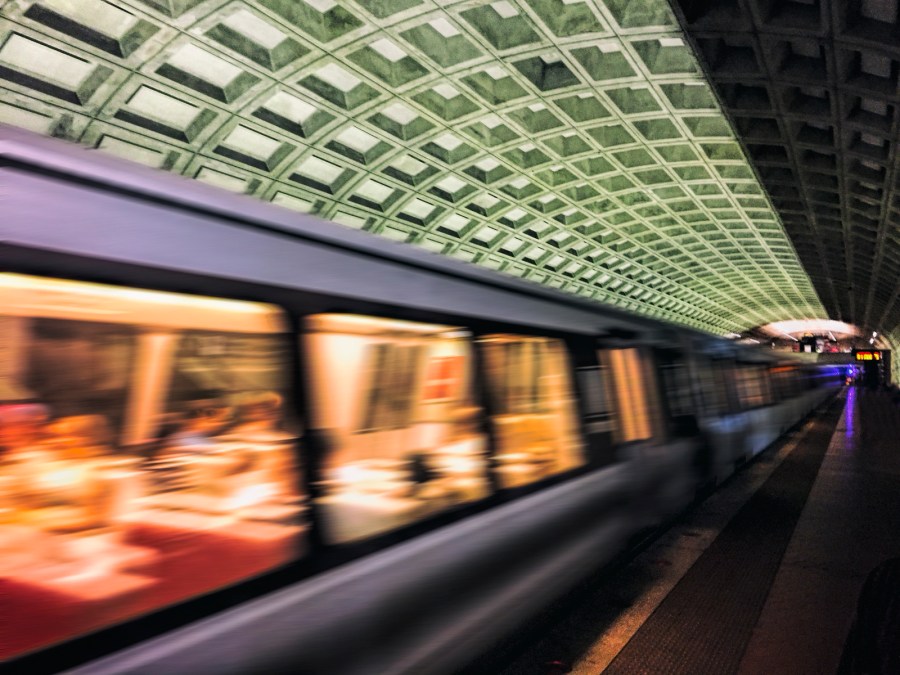
Virginia Tech’s Urban Computing Program has partnered with local cities to help industry and governments short on data management resources develop data-driven solutions to their urban challenges, Naren Ramakrishnan, UrbComp director, said during a presentation in Washington D.C. last week.
The growth of urban populations can cause challenges in transportation, public health and city services, but it also greatly increases the amount of city data available. Virginia Tech students are in turn able to analyze datasets and create algorithms that can help solve the growing problems faced by urban areas. This practice is known as “urban computing.”
Virginia Tech’s UrbComp program, funded by a nearly $3 million grant from the National Science Foundation Research Trainee Program, trains students in the latest methods for analyzing massive datasets and teaches key issues surrounding growing urban populations. Nine graduate students are currently sponsored to participate in the program and are partnered with industry and government organizations so they can apply their learning to the real world, Ramakrishnan said.
With limited resources and talent, some cities are unable to effectively use the operational and behavioral data they collect, Ramakrishnan said. Organizations without the capacity to develop and implement data-supported solutions, he said, are able to rely on the expertise of UrbComp’s student team to leverage data-driven problem solving.
The UrbComp team collaborates with industry and government groups and has worked on several projects involving Washington D.C.’s metro system. Students created algorithms to detect oncoming traffic through real-time social media analysis and to predict train performance using passenger data.
In partnering with the Washington Metropolitan Area Transit Authority last year, the UrbComp team asked metro administrators what problems they wanted to solve first.
“They said something that was music to our ears,” Ramakrishnan said. “They said,’we have lots of problems.'”
Using anonymized data on the flow and travel times of passengers and train-to-station interactions, Ramakrishnan said “students developed ‘deep learning’ techniques to map passenger data.” The deep learning algorithms were able improve based on past data and thereby predict, with some reliability, trains’ on-time performance, she said. Ramakrishnan said this high-level analysis of transportation data will eventually allow WMATA to better estimate when and where delays will occur, though the algorithm is not yet being used by the agency to alert passengers of delays.
Other projects looked at the effects of rezoning based on population and school district data and another looked at complex crime data with the goal of mitigating prejudice in law enforcement. The team has also worked with the D.C.-area Loudoun County Public Schools district.